Non-invasive Glucose Sensing
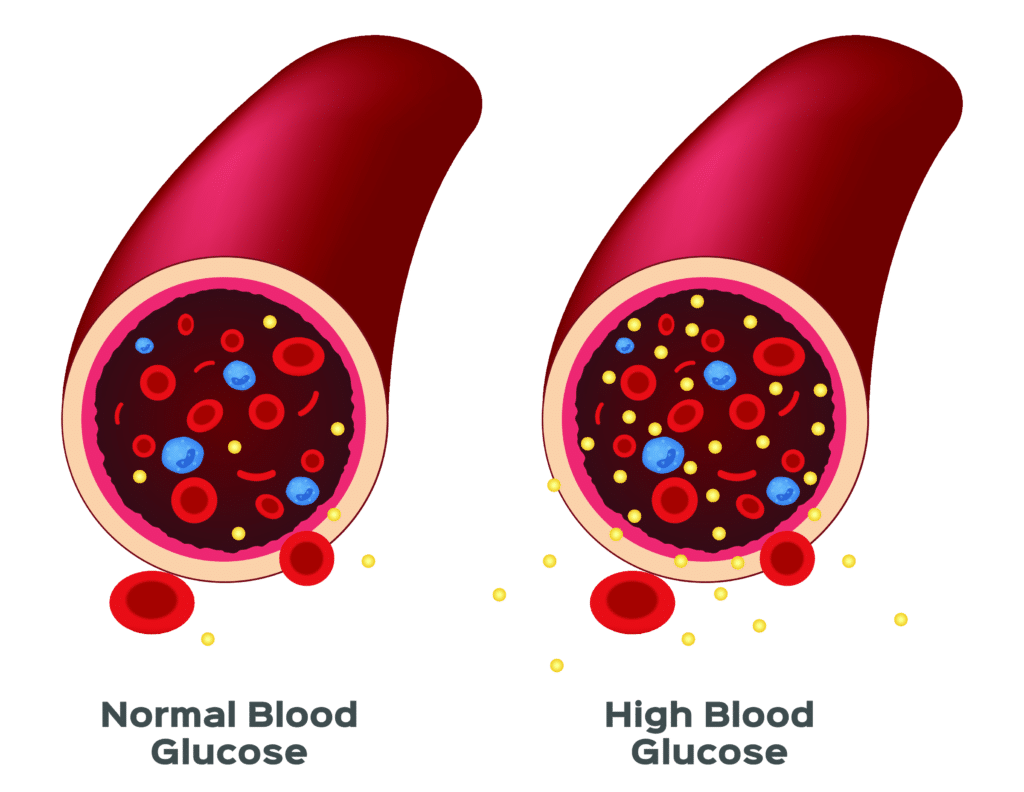
Diabetes is a disease that impacts a significant percent of people around the world. Patients with diabetes often need to measure their blood glucose multiple times a day, primarily by puncturing their finger to fetch a droplet of blood thereby causing pain and skin injury. Our goal is to develop a non-invasive mobile device that can accurately and robustly monitor glucose concentration.
In addition, this project has now received NSF funding (award #: 2037267) to develop and pilot a joint multimodal sensing system that can provide continuous noninvasive glucose monitoring data in addition to automated meal detection with an estimate of meal intake. Both types of information are necessary to inform artificial pancreas algorithms for prediction of bolus (i.e. short-acting) insulin dose needs. More specifically, the proposed system is in the form of an ear-worn device will tackle key systems and algorithmic challenges to realize a fully closed-loop artificial pancreas system. The intellectual merits of the proposed work are following. First, it will develop novel system designs to realize fine-grained, robust dietary sensing by fusing three streams of signals (i.e., bio-electrical signals, motion, and acoustic signals); Second, it will advance existing optical-based noninvasive glucose monitoring by addressing challenges of skin scattering and mitigating the impact of confounding factors and user diversity; Third, it will present new energy-efficient machine learning models dedicated to enabling local execution at micro-controllers, dealing with the limited number of labeled training data, and ensuring data privacy and communication efficiency; Finally, it will characterize and validate biological and behavioral biomarkers most relevant to diabetes management for implementing an artificial pancreas systems and develop new approaches for adaptive sampling and noise handling to ensure accurate monitoring of target events.