Engineering in Medicine and Biology Conference (EMBC), 2022
Investigating Temporal Patterns of Glycemic Control around Holidays
Prajakta Belsare, Baiying Lu, Abigail Bartolome, Temiloluwa Prioleau
Published
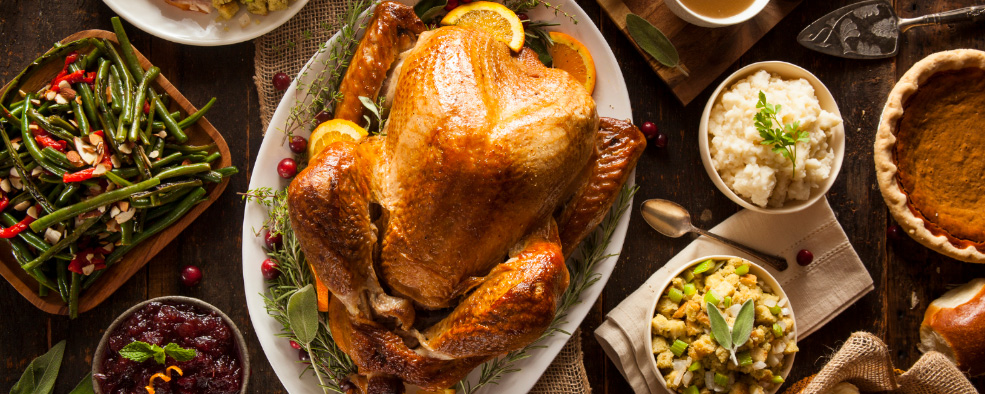
Image Source
https://www.cdc.gov/foodsafety/communication/holidays.html